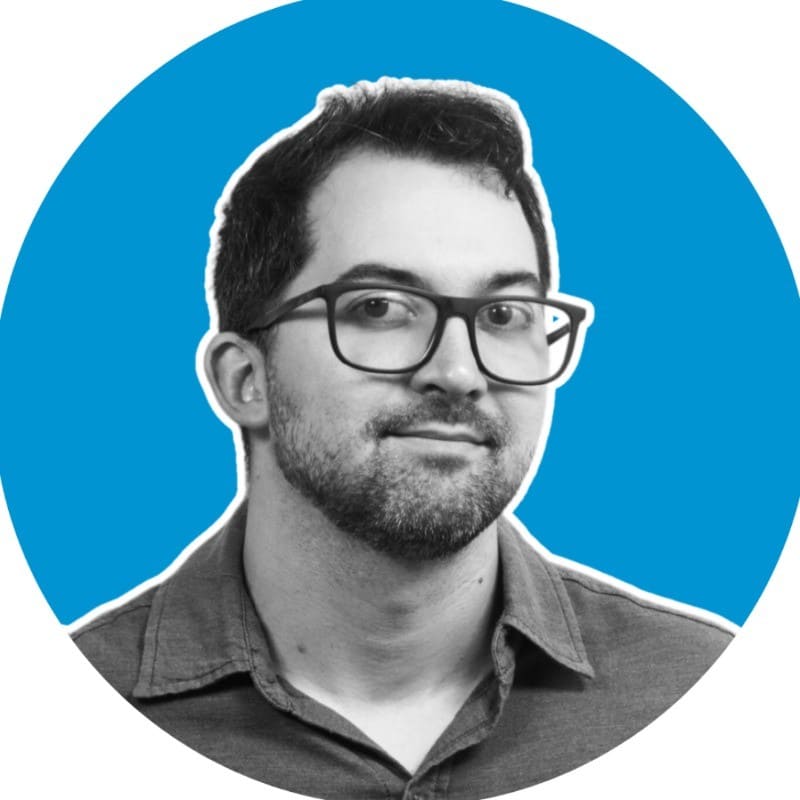
By
Raphael Santos
September 24, 2021
Updated
September 20, 2023
Source: iStock/Andy
Artificial intelligence (AI) is transforming societies and economies. It enhances the speed, efficiency and precision of human efforts and is being adopted across most industries.
However, according to a survey by the European Commission, businesses feel that access to the right skill sets is the biggest impediment to adopting AI.
As the artificial intelligence workforce continues to expand, it’s important for tech professionals to consider honing their skills in certain areas if they want to pursue a career in AI.
With the rapid rise in the adoption of AI, professionals must grasp their significance in this ever-changing field. Managing data has become a top priority for businesses globally, as evidenced in the chart below.
All professionals must be equipped with AI skills to handle new tasks effectively. Knowing which skills are the most in demand can help candidates work out what they need to develop for roles such as machine learning engineer or data scientist.
- Types of AI
1.1. Machine learning
1.2. Deep learning
1.3. Neural network architectures
1.4. Computer vision - Programming languages and intelligence skills
2.1. Python
2.2. R
2.3. Java
2.4. C++ - Libraries and frameworks
3.1. TensorFlow
3.2. NumPy
3.3. SciPy
3.4. Scikit-learn
3.5. PyTorch
3.6. Apache Spark - Continual AI skills development
- Domain knowledge
- The emergence of ChatGPT and the skills needed
6.1. The impact of Generative AI in business - Soft skills
7.1. Communication
7.2. Leadership
7.3. Ability to work as a team
7.4. Creativity
7.5. Analytical thinking - Find your next AI job with Airswift
Read on to find out more about the key AI skills in demand.
Types of AI
Here are the various types of AI to develop skills in:
Machine learning
Machine learning is a subset of AI that allows systems to automatically learn and improve from experience without explicit programming. Machine learning uses different algorithms, such as neural networks (which we’ll discuss later) to solve problems.
Deep learning
Deep learning is a specific type of machine learning that imitates the way humans obtain particular knowledge. It is an important component of data science and includes statistics and predictive analytics. Examples of deep learning include face recognition, virtual assistants and vision enablement for driverless cars.
Neural network architectures
Neural networks make up part of the deep learning process and are inspired by the structure of the human brain. They are complex structures created from artificial neurons that can process multiple inputs and produce a singular output. Understanding this architecture is essential for deep learning.
Computer vision
Computer vision involves training computers to understand and interpret the visual world. This includes accurately identifying and locating objects, then reacting to what they see through the use of digital images from deep learning models, videos and cameras.
Programming languages and intelligence skills
Source: iStock/gorodenkoff
Learning specific programming languages is essential for work in the AI field. Python, R, Java and C++ are the most commonly sought after.
Python
Python is one of the most popular programming languages in use at the moment. It was created in 1991, and has since been one of the most widely used programming languages, alongside Java and C++.
It is useful for AI because it is reliable, simple to use and increases execution time. Python requires the least code compared to other languages and has pre-built libraries for scientific computation, advanced computing and machine learning.
R
R is commonly used in new-style AI that involves statistical computations, machine learning, numerical analysis, neural networks and the use of Bayesian inference.
This language allows you to gather and clean up datasets, use linear algebra and matrix transformations to process data, and apply statistical and machine learning functions.
HackerRank has an online certification course to teach you how to use R.
Java
Java is another of the most commonly used programming languages for AI projects. It is used mostly for AI intelligence programming, machine learning solutions, multi-robot systems, genetic programming, neural networks and search algorithms.
You can learn Java programming on the HackerRank site.
C++
One of the oldest and fastest programming languages, C++ can manipulate hardware resources and provide procedural programming for CPI intensive applications. It can be used to develop browsers, operating systems and games. C++ supports various programming methods such as procedural, functional and object-oriented functions, making it a very flexible programming language.
Libraries and frameworks
Besides programming languages, you need to be able to understand and apply the following concepts and techniques in your work, regardless of programming language.
TensorFlow
TensorFlow is an open source platform for machine learning that provides a wealth of resources, tools and libraries to enable developers to build machine learning applications.
It was built by Google with the C++ programming language, but coders can also use Python when developing applications for this AI engine.
NumPy
NumPy is a fundamental package for scientific computing in Python. It facilitates advanced mathematical operations on large data sets, which are otherwise typically executed with less code than is possible within Python’s existing sequences.
NumPy provides a multidimensional array object and variations like matrices and masks. These can be used for a number of mathematical operations.
SciPy
An open-source library within Python, SciPy is used to solve mathematical and scientific problems. It is built upon the NumPy extension and enables users to visualise and manipulate data with a variety of high-level commands.
Scikit-learn
Scikit-learn is considered one of the most robust and useful libraries for machine learning in Python. It includes a plethora of tools for statistical modelling and machine learning, such as dimension reduction, classification, clustering and regression, all through a consistent interface in Python.
PyTorch
PyTorch is most commonly used for deep learning applications using CPUs and GPUs. It is an open source machine learning library for Python, developed predominantly by the Facebook AI research team.
Apache Spark
Apache Spark is a distributed, open source processing system that is used for big data workloads. It uses optimised query execution and in-memory caching for analytic queries against all sizes of data.
Continual AI skills development
It is also important to highlight the need to constantly improve existing knowledge and learn new technologies when working in AI. A survey by Stack Overflow asked developers how frequently they learn a new language or skill, and 75% said they do this once every few months or once a year.
This shows us how even the best candidates need to adapt and learn constantly to keep up.
Areas that are useful to develop include brand new programming languages such as Triton and Julia, as well as more established languages like GEM, which was established in 1985.
Domain knowledge
Having specialist technical knowledge and expertise in machine learning and artificial neural networks is a good start to becoming an ML engineer, but many companies expect more.
For example, engineers should have domain experience in their business. This knowledge helps them to understand the specifics of the industry and any challenges the business is trying to resolve.
The emergence of ChatGPT and the skills needed
The rise of sophisticated conversational agents like ChatGPT has led to an increasing demand for a unique skill set that merges traditional software engineering with machine learning and natural language processing (NLP) expertise.
Proficiency in Python, experience with cloud-based services like AWS or Azure, and an understanding of machine learning frameworks such as TensorFlow or PyTorch are foundational skills.
In addition, a deep understanding of NLP techniques, ranging from basic text processing to more complex tasks like sentiment analysis, is crucial for refining and customizing these agents for specific business applications.
Moreover, data science skills, especially data pre-processing and analysis, are essential in training and fine-tuning the machine learning models that form the core of these chatbots.
The impact of Generative AI in business
The impact of ChatGPT on businesses is likely to grow exponentially in the coming years as the technology matures and as companies increasingly realize the value of AI-driven interaction.
Beyond cost-saving measures, the adaptability of these systems allows for their use in a broad range of applications, from sales and marketing to HR and internal communications.
As data analytics capabilities advance, these chatbots will not only handle queries but will also predict customer needs and make proactive suggestions, thereby turning them into not just responsive but also predictive tools for businesses.
Thus, companies that invest in this technology and the requisite skills for its implementation are positioning themselves for more efficient operations and a stronger competitive edge.
Soft skills
Source: iStock/gradyreese
In addition to the technical skills necessary to work in AI engineering, it is also essential to have soft skills that complement that technical knowledge.
A machine can better perform repeatable, rote tasks. But what happens when something goes wrong? Where thinking on the fly is needed, humans still have the upper hand.
Just as a pilot’s main role is to monitor the autopilot, they’re still needed to step in if there’s a problem. Then there’s the benefit of retaining rare expertise and experience, and the fact that sometimes it’s more cost effective to augment a human role with robots than completely automate it.
It’s more often specific tasks rather than entire jobs that are automated. Robots need a human touch like these:
Communication
This means being able to accurately and clearly communicate ideas across the business, using the correct tone and body language to deliver your messaging.
Take the automotive sector as an example that has automated its manufacturing processes for years. Different production stages are broken down into smaller, repeatable tasks. However, errors can still occur. If staff fail to flag these issues to each other, they slip through the cracks and lead to problems in the final product.
For the oil and gas sector, AI does a great job in speeding up processes. But with so many moving parts on a given project, all team members need to keep each other updated in order for it to function smoothly.
Leadership
According to the Harvard Business Review, almost 50 years of meta-analytic studies regard emotional characteristics twice as important as IQ to be an effective leader. These characteristics include emotional stability, extroversion and curiosity.
This shows it’s in the very most human aspects of our characters that we possess the capacity to lead. Machines can compute faster than the human mind, but can they read a situation and know the right words to motivate a team? These softer characteristics go a long way towards separating man from machine.
Ability to work as a team
Engineers will also need to be able to manage teams where necessary, as well as work closely with other teams (such as robotics, electrical engineering and software engineering) to run and implement AI projects.
Furthermore, it is essential for every worker to possess a high level of adaptability, being open to learning new things and being readily available to assist their colleagues. This demand for adaptability reflects the ever-changing nature of the field and the importance of collaboration within the team.
New technologies will require continuous learning. For businesses, there must be an active attempt to identify those in highest demand and implement relevant training procedures around them.
Creativity
AI requires new ways of thinking, which means creativity is an essential skill for engineers in this field.
Creativity enables them to explore uncharted territories, experiment with new algorithms, and envision groundbreaking applications for AI technology.
Moreover, creativity plays a crucial role in the development of user-friendly and intuitive AI interfaces. Engineers with a creative mindset can design visually appealing and interactive interfaces that effectively communicate with users and provide seamless experiences.
By incorporating elements of design thinking and user-centered design, they can create AI systems that are not only technically advanced but also aesthetically pleasing and user-friendly.
Analytical thinking
Critical thinking skills allow engineers to come up with innovative ideas and solve complex problems using logic and reasoning.
In the age of automation, the value simply doesn’t lie in the data you collect. It’s in the way you use it to achieve your end goal. For the energy sector, the onus is now on developing problem-solving strategies to help companies tackle these issues.
Want more insight into talent trends in the technology industry? Click the link below to download our latest whitepaper.
Find your next AI job with Airswift
Here at Airswift, we have more than 40 years of experience in helping candidates find jobs with some of the world’s biggest companies.
With our unrivalled global presence, understanding of tax regulations, labour and payroll, we can support you at every stage of the recruitment process.
Get started today! Register with our job board and set up alerts for notifications tailored to your job search.